Article
Is data supply AI’s Achilles’ heel?
AI needs data to keep on improving, but the supply is vulnerable to disruption. There is an imbalance of benefits between the companies who monetize data and the rest of society who produces it, which needs addressing.
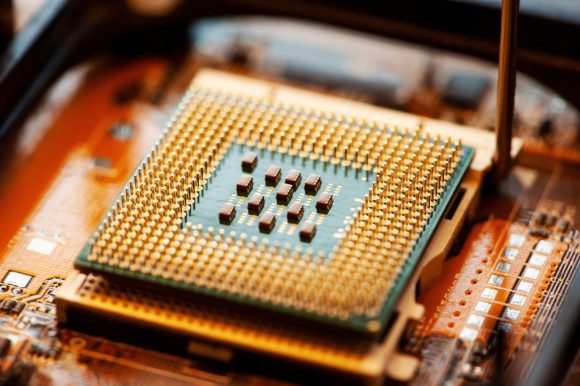
AI relies on a large, ready supply of data, but there is a risk that AI itself starts to threaten that supply’s quantity and quality. Currently, large AI models are being trained on data that has often not been provided willingly but scraped from various sources, disregarding copyright concerns.1 This benefits a relatively small group of technology companies who are equipped to collect, process, and model data at scale, while those “data laborers” (Box 1) who produce the data—not just professional “content creators” but all of us who generate data through our use of, or interaction with digital content or services of any sort—receive little or no remuneration or say in how it is used.2
AI’s reliance on such sources is a vulnerability, which is compounded by the fact that the creators of the data on which AI relies are many of the same ones exposed to AI, through automation, impersonation, or manipulation. This is not a sustainable paradigm. Maintaining a ready flow of data must be in everyone’s interests if it is not to be vulnerable to disruption.
Box 1: What is data labor?
Box 1: What is data labor?
The “data labor” concept has been defined as “activities that produce digital records useful for capital generation,”3 i.e., production of data capital.4 The definition is as broad as the content that can be used to train AI—behavior logs of social media use, personal information, labelled datasets, digitized media content like articles, books, films, and photos, all are arguably products of data labor.
How could the flow of data be disrupted? The most obvious way is by “data strikes”—data laborers no longer using certain services, demanding their data be deleted where possible, like in the EU, or by using tools to prevent data collection, like ad blockers and anti-tracking browser extensions.5 Another, more aggressive route, is data “poisoning,” e.g., changing pixels in artworks in ways that disrupt a model’s training when scraped.6 Another might be to selectively contribute data to one organization over another.
The above forms of data disruption have all taken place in practice-the question is whether they reach a scale that disrupts the broader data ecosystem. Why the concern now, when big tech companies have arguably “got away” with monetizing users’ data for years? The difference is that where they were using the data primarily to sell advertising, AI could be used to undercut or replace “real” (human) labor, without adequate compensation. Business models that rely on this imbalance look vulnerable to backlash at multiple levels, from users, to regulators, to rivals that make efforts to align incentives with data laborers, rather than just exploit them. This vulnerability is likely to be exacerbated further as “natural” (i.e., non-synthetic) data shortages potentially start to bite from around 2026.7
Is synthetic data a potential solution?
Is synthetic data a potential solution?
Synthetic data could help, but overreliance on it has been shown to degrade models if outputs are used for multiple training iterations, eventually leading to model collapse.8 Maintaining the supply of “natural” data should be a priority for AI firms, as well as for wider society, which has an interest in improving the capabilities of AI models, assuming the right safeguards are in place.
There are three categories of solution to be considered:
- Bilateral: Direct agreements between data producers and technology, like the licensing agreements between Google and social media site Reddit, or OpenAI and the Financial Times.
- Multilateral: Agreements between tech companies and coalitions or unions of data producers, e.g., Streamr.
- Societal: Agreements at the societal level can be either imposed on tech companies, e.g., by regulations; or negotiated, e.g., by government bodies like the National Health Service.
In practice, a mixture of all three of the above avenues will likely need to be explored. While there is a risk that this shifts us from a freer data world to a more restricted one, what we currently have is more akin to a free rider problem. Data that has a monetary value is being used in many cases for free by those monetizing it. Shifting that balance in favor of data producers should help to safeguard the supply of data by improving incentives to produce it and make it available, contributing to a healthy data ecosystem, which is to everyone’s benefit.
The author thanks the following for their input: Mike Ryan, Jackie Bauer, Richard Mylles, William Nicolle.
For further information, please visit www.ubs.com/institute-disclaimer